October 25–27, 2023
NCSA Headquarters, University of Illinois Urbana-Champaign
Generative AI refers to a wide variety of artificial intelligence and machine learning tools capable of producing content such as images, videos, text and computer programs. The emergence of publicly available products such as ChatGPT, Bard, Dall-E, and Stable Diffusion has the potential to enhance human productivity dramatically while also posing important questions regarding their ethical use in society.
The workshop brings together experts to discuss the fundamental science and technology behind the myriad of tools collectively known as Generative AI, and discuss their societal applications and challenges.
RECORDINGS
Watch videos of all presentations as linked below, on the Generative AI Workshop playlist, and on the C3.ai DTI YouTube Channel homepage (scroll down to Workshops).
ORGANIZERS
Julia Hockenmaier, (University of Illinois Urbana-Champaign), Alex Schwing (University of Illinois Urbana-Champaign), R. Srikant (University of Illinois Urbana-Champaign), Tandy Warnow (University of Illinois Urbana-Champaign)
SPEAKERS
Mikhail Belkin (University of California, San Diego), Stevie Chancellor (University of Minnesota), Edward Delp (Purdue University), Shalini De Mello (NVIDIA), David Forsyth (University of Illinois Urbana-Champaign), Abhinav Gupta (Carnegie Mellon University), Tatsunori Hashimoto (Stanford University), Karrie Karahalios (University of Illinois Urbana-Champaign), Jian Ma (Carnegie Mellon University), Morteza Mardani (NVIDIA), Henrik Ohlsson (C3 AI), Hao Peng (University of Illinois Urbana-Champaign), Max Raginsky (University of Illinois Urbana-Champaign), Alex Schwing (University of Illinois Urbana-Champaign), Greg Shakhnarovich (Toyota Technological Institute at Chicago), Shenlong Wang (University of Illinois Urbana-Champaign), Yuxiong Wang (University of Illinois Urbana-Champaign), Ben Zhao (University of Chicago)
PROGRAM
Day 1 (Wednesday, October 25)
Session Chairs:
Morning: Varun Chandrasekaran (University of Wisconsin-Madison)
Afternoon: Vlad Kindratenko (University of Illinois Urbana-Champaign) and Bruno Abreu (University of Illinois Urbana-Champaign)
ABSTRACT
I describe recent work, joint with Anand Bhattad, that shows that generative image models “know” much more than anyone expected. In particular, it is quite straightforward to force a StyleGAN model to produce multiple different lightings of a scene. Startlingly, one can force it to produce albedo maps, depth maps, and normal maps — things we know are useful for rendering, but didn’t expect to find lying about inside an image generator. I describe evidence that stable diffusion models “know” much more than one would expect, too.
SPEAKER
David Forsyth is the Fulton Watson Copp Chair in Computer Science at the University of Illinois Urbana-Champaign. He received the BSc and MSc degrees in electrical engineering from the University of the Witwatersrand, Johannesburg, and the MA and DPhil degrees from Oxford University. He has published more than 170 papers on computer vision, computer graphics, and machine learning. He has served as program co-chair or as general co-chair for numerous major computer vision conferences. He became an ACM fellow in 2014. His textbook, Computer Vision: A Modern Approach (joint with J. Ponce, published by Prentice Hall) is widely adopted as a course text. A further textbook, Probability and Statistics for Computer Science, came out in 2021; his latest, Applied Machine Learning, this year. He has served two terms as editor in chief, IEEE Transactions on Pattern Analysis and Machine Intelligence, serves on a number of scientific advisory boards, and has an active practice as an expert witness.
ABSTRACT
Recent years have seen significant advances in robot learning. However, the data still remains the biggest bottleneck when it comes to robot learning. It is significantly hard to collect large-scale and diverse physical data in the wild. In this talk, I discuss how we can overcome the data barrier in robotics by exploiting the passive data streams: videos of humans performing actions. I introduce how we can use large-scale videos to learn meaningful visual representations. These representations capture the state vectors and can be used to learn meaningful policies. Next I address how we can use these passive videos to learn how to manipulate objects themselves. Here we use the videos to learn functional distances that help us learn rewards for learning policies. Finally, we try to answer the question: What is a good dataset in robotics? We perform large-scale experiments to understand the property of what makes a good dataset in robotics.
SPEAKER
Abhinav Gupta is an Associate Professor at the Robotics Institute, Carnegie Mellon University. His research focuses on scaling up learning by building self-supervised, lifelong and interactive learning systems. Specifically, he is interested in how self-supervised systems can effectively use data to learn visual representation, common sense and representation for actions in robots. Abhinav is a recipient of several awards including IAPR 2020 JK Aggarwal Prize, PAMI 2016 Young Researcher Award, ONR Young Investigator Award, Sloan Research Fellowship, Okawa Foundation Grant, Bosch Young Faculty Fellowship, YPO Fellowship, IJCAI Early Career Spotlight, ICRA Best Student Paper award, and the ECCV Best Paper Runner-up Award. His research has also been featured in Newsweek, BBC, Wall Street Journal, Wired and Slashdot.
ABSTRACT
In the rapidly evolving landscape of AI, a transformative shift from content retrieval to content generation is underway. Central to this transformation are diffusion models, wielding remarkable power in visual data generation. My talk touches upon the nexus of generative AI and NVIDIA’s influential role therein. I navigate through diffusion models, elucidating how they establish the bedrock for leveraging foundational models. An important question arises: How to integrate the rich prior of foundation models in a plug-and-play fashion for solving downstream tasks, such as inverse problems and parametric models? Through the lens of variational sampling, I present an optimization framework for sampling diffusion models that only needs diffusion score evaluation. Not only does it provide controllable generation, but the framework also establishes a connection with the well-known regularization by denoising (RED) framework, unveiling its extensive implications for text-to-image/3D generation.
SPEAKER
Morteza Mardani is a Senior Research Scientist at NVIDIA focusing on generative learning. Concurrently, he is a visiting researcher within the Electrical Engineering department at Stanford University. Morteza’s journey includes previous appointments as a postdoctoral researcher and research associate at Stanford, and visiting scholar at the RISE Lab at UC Berkeley. Morteza received his PhD from the University of Minnesota, EE department, in May 2015. His contributions to large-scale data science were recognized with the Young Author Best Paper Award from the IEEE Signal Processing Society in 2017.
ABSTRACT
In this talk, based on joint work with Belinda Tzen and with Tanya Veeravalli, I discuss theoretical foundations of generative modeling and function approximation using diffusion models. I introduce a unified viewpoint on both sampling and variational inference in these models through the lens of stochastic control. Building on these ideas, I demonstrate that one can efficiently sample from a wide class of terminal target distributions by choosing the drift of the latent diffusion from the class of multilayer feedforward neural nets, with the accuracy of sampling measured by the Kullback-Leibler divergence to the target distribution. I also discuss the relation between the expressive power of diffusion-based function approximators and nonlinear controllability, i.e., the problem of optimally steering a certain deterministic dynamical system between two given points in finite time. I conclude with an outline of ongoing work and future directions.
SPEAKER
Maxim Raginsky received the B.S. and M.S. degrees in 2000 and the Ph.D. degree in 2002 from Northwestern University, all in Electrical Engineering. He has held research positions with Northwestern, the University of Illinois Urbana-Champaign (where he was a Beckman Foundation Fellow from 2004 to 2007), and Duke University. In 2012, he has returned to UIUC, where he is currently a Professor with the Department of Electrical and Computer Engineering and the Coordinated Science Laboratory. He also holds a courtesy appointment with the Department of Computer Science.
ABSTRACT
Remarkable recent advances in deep neural networks are rapidly changing science and society. Never before had a technology been deployed so widely and so quickly with so little understanding of its fundamentals. I argue that developing a fundamental mathematical theory of deep learning is necessary for a successful AI transition and, furthermore, that such a theory may well be within reach.
I discuss what such a theory might look like and some of its ingredients that we already have available. In particular, I discuss why infinitely wide neural networks make sense from both theoretical and practical points of view, and how feature learning can be incorporated into the resulting algorithms.
SPEAKER
Mikhail Belkin is a Professor of Computer Science and Engineering at the University of California, San Diego. He received his Ph.D. in 2003 from the Department of Mathematics at the University of Chicago. His research interests are in theory and applications of machine learning and data analysis. Some of his well-known work includes widely used Laplacian Eigenmaps, Graph Regularization, and Manifold Regularization algorithms, which brought ideas from classical differential geometry and spectral analysis to data science. His recent work concerns understanding remarkable mathematical and statistical phenomena observed in deep learning. This empirical evidence necessitated revisiting some of the basic concepts in statistics and optimization. One of his key recent findings is the “double descent” risk curve that extends the textbook U-shaped bias-variance trade-off curve beyond the point of interpolation. Mikhail Belkin is a recipient of a NSF Career Award and a number of best paper and other awards. He has served on the editorial boards of the Journal of Machine Learning Research, IEEE Pattern Analysis and Machine Intelligence and SIAM Journal on Mathematics of Data Science.
ABSTRACT
Generative AI models have changed the way we produce and consume content. In the absence of regulations on ethical data acquisition and training, rampant misuse of GenAI tools has produced widespread harm on some of the most vulnerable segments of the workforce: artists. I discuss some of negative impacts on human creatives in multiple industries, particularly artists, and describe adversarial ML tools developed to mitigate these harms. I describe experiences and lessons learned from deploying an adversarial ML tool (Glaze) at scale.
SPEAKER
Ben Zhao is a Neubauer Professor of Computer Science at University of Chicago. He completed his Ph.D. at UC Berkeley (2004) and B.S. from Yale (1997). He is a Fellow of the ACM, and a recipient of the NSF CAREER award, MIT Technology Review’s TR-35 Award (Young Innovators Under 35), USENIX Internet Defense Prize, ComputerWorld Magazine’s Top 40 Technology Innovators award, IEEE ITC Early Career Award, and Faculty awards from Google, Amazon, and Facebook. His work has been covered by many media outlets including New York Times, CNN, NBC, BBC, MIT Tech Review, Wall Street Journal, Forbes, and New Scientist. He has published over 180 articles in areas of security and privacy, machine learning, networking, and HCI. He served as TPC (co-)chair for the World Wide Web conference (WWW 2016) and ACM Internet Measurement Conference (IMC 2018). He also serves on the steering committee for HotNets.
ABSTRACT
I present an overview of the current state of generated and manipulated media, such as Deep Fakes, and describe how these methods work, where they are being used, and how to detect them. I also describe the history of manipulated media content and how we got where we are today. A synopsis:
Historical Overview of Manipulated Media. The disappearing Russians. Hollywood and CGI. Cheapfakes and Deepfakes. Seeing is not believing. An overview of the technology. The threat of manipulated media. Where is this all going in 5, 10, 20 years? Is help coming?
SPEAKER
Edward J. Delp is the Charles William Harrison Distinguished Professor of Electrical and Computer Engineering, Professor of Biomedical Engineering, and Professor of Psychological Sciences (Courtesy) at Purdue University. His research interests include image and video processing, image analysis, computer vision, image and video compression, multimedia security, medical imaging, multimedia systems, communication and information theory. He has published and presented more than 500 papers. He is a Fellow of the IEEE, a Fellow of the SPIE, a Fellow of the Society for Imaging Science and Technology (IS&T), and a Fellow of the American Institute of Medical and Biological Engineering.
ABSTRACT
My work is about community-centered interaction and community-centered computing. From this framing, this talk covers how people have operationalized such algorithmic systems, what we should do before we publicly release such systems, and what mechanisms to put in place to monitor such systems. We examine automated educational systems, the use of which spiked during the pandemic, and their impacts.
SPEAKER
Karrie Karahalios is an Assistant Professor of Computer Science at the University of Illinois Urbana-Champaign where she heads the Social Spaces Group. Her work focuses on the interaction between people and the social cues they perceive in networked electronic spaces. Of particular interest are interfaces for pubic online and physical gathering spaces such as chatrooms, cafes, parks, etc. The goal is to create interfaces that enable users to perceive conversational patterns that are present, but not obvious, in traditional communication interfaces.
Karrie completed a S.B. in electrical engineering, an M.Eng. in electrical engineering and computer science, and an S.M. and Ph.D in media arts and science at MIT.
SPEAKER
Kratika Bhagtani (Purdue University), FGSSAT : Unsupervised Fine-Grain Attribution of Unknown Speech Synthesizers Using Transformer Networks
Jiahao Li (Toyota Technological Institute at Chicago), Instant3D: Fast Text-to-3D with Sparse-View Generation and Large Reconstruction Model
Jipeng Lyu (University of Illinois Urbana-Champaign), NeuralEditor: Editing Neural Radiance Fields via Manipulating Point Clouds
Logan Stapleton (University of Minnesota), Using Large Language Models as Virtual Patients for Suicide Prevention Training
Haochen Wang (Toyota Technological Institute at Chicago), Score Jacobian Chaining: Lifting Pretrained 2D Diffusion Models for 3D Generation
Ziyang Xie (University of Illinois Urbana-Champaign), MV-Map: Offboard HD-Map Generation with Multi-view Consistency
Amit Kumar Singh Yadav (Purdue University), DSVAE: Interpretable Disentangled Representation for Synthetic Speech Detection
Xiaocong Yang (University of Illinois Urbana-Champaign), Parameter-Efficient Tuning with Special Token Adaptation
Andy Zhou (University of Illinois Urbana-Champaign), Language Agent Tree Search Unifies Reasoning Acting and Planning in Language Models
Libin Zhu (University of California, San Diego), Quadratic Models for Understanding Neural Network Dynamics
Day 2 (Thursday, October 26)
Session Chairs:
Morning: Aaron Saxton (University of Illinois Urbana-Champaign) and Xiaoxia Liao (University of Illinois Urbana-Champaign)
Afternoon: George Heintz (University of Illinois Urbana-Champaign)
ABSTRACT
Instruction-following language models have driven remarkable progress in a range of NLP tasks and have seen rapid adoption by end users. However, studying these models has been challenging, due to the lack of replicable, academic studies and the high costs of data collection. This talk outlines a few of our recent works, such as AlpacaFarm, that leverage large language models to enable low-cost, fast, and replicable experiments in studying and improving instruction following language models.
SPEAKER
Tatsunori Hashimoto is an Assistant Professor of Computer Science at Stanford University. His research uses tools from statistics to make machine learning systems more robust and reliable, especially in challenging tasks involving natural language. His research is designed to use robustness and worst-case performance as a lens to understand and make progress on several fundamental challenges in machine learning and natural language processing.
Previously, Hashimoto was a post-doc at Stanford working for John C. Duchi and Percy Liang on tradeoffs between the average and worst-case performance of machine learning models. Before that, he was a graduate student at MIT co-advised by Tommi Jaakkola and David Gifford, and an undergraduate student at Harvard in statistics and math advised by Edoardo Airoldi.
ABSTRACT
Publicly available large language models (LLMs) such as Llama have substantially accelerated the innovations of the open-source community. It takes a surprisingly small amount of finetuning to elicit impressive capabilities from strong pretrained models, spawning hundreds of finetuned variants. The community’s collective effort has positioned it strongly to close the gap between open-source and proprietary LLMs. Bold claims have been made, often justified by impressive performance on established benchmarks. There are, however, reasons to pause. In the first part of this talk, I argue that the gap between open-source and proprietary models is, unfortunately, larger than many evaluations suggest. This observation stems from our recent evaluation framework addressing models’ capabilities of learning from feedback in multiturn exchanges, a setting that better reflects real-world applications than many established benchmarks. Our findings reveal new insights into existing models and highlight previously overlooked opportunities. In the second part, I delve deeper into learning from feedback, and investigate today’s LLMs’ potential to continuously improve themselves through self-play, without direct human intervention. The findings of both parts highlight the challenges of properly evaluating LLMs, and suggest the necessity of adapting our evaluation protocols to our ever-stronger models. I close with our ongoing effort to build a crowdsourced evaluation platform to facilitate the continuously evolving evaluation of LLMs.
SPEAKER
Hao Peng is an Assistant Professor at the Department of Computer Science of the University of Illinois Urbana-Champaign. His research interest spans natural language processing and machine learning. His current interests primarily include making language AI more efficient and accessible, and evaluating and improving large-scale language models’ reasoning capabilities, factuality, and trustworthiness.
ABSTRACT
Computer vision and machine learning have been remarkably successful in the last 10 years. Models that analyze work very well. But predicting what you observe is not sufficient any more. You want to observe what’s behind an image, or have forecasting ability – to look beyond what is visible. To do that, you need many systems to work well together. I provide background on generative modeling, and evaluate different types of generative models, their distinctions, advantages, and disadvantages.
SPEAKER
Alex Schwing is an Associate Professor in the Department of Electrical and Computer Engineering at the University of Illinois Urbana-Champaign and affiliated with the Coordinated Science Laboratory and the Computer Science Department. Prior to that, he was a postdoctoral fellow in the Machine Learning Group at University of Toronto collaborating with Raquel Urtasun, Rich Zemel, and Ruslan Salakhutdinov. Alex completed his PhD in computer science in the Computer Vision and Geometry Group at ETH Zurich working with Marc Pollefeys, Tamir Hazan, and Raquel Urtasun, and graduated from Technical University of Munich (TUM) with a diploma in Electrical Engineering and Information Technology. Alex’s research is centered around machine learning and computer vision. He is particularly interested in algorithms for prediction with and learning of non-linear (deep nets), multivariate and structured distributions, and their application in numerous tasks (e.g., for 3D scene understanding from a single image).
ABSTRACT
Humans are remarkably adept at visual recognition in the wild. We recognize many thousands of object categories; at all levels of recognition: pixel, group and image; and quickly learn new categories with very few labeled examples. However, AI algorithms lag significantly behind and are limited to far smaller closed vocabularies of hundreds of object categories, especially for pixel-level labeling tasks. How can we scale AI systems to human-like recognition capabilities? Recent image-text foundation models, e.g., CLIP, which are trained on large Internet-scale data, present a promising path towards improving image-level zero-shot recognition. Going beyond image recognition, we present our pioneering work on exploring the more challenging problem of pixel-level open-vocabulary recognition, with large text-image contrastive and generative foundational models. We present the learnings that we’ve garnered from our work in terms of the capabilities and challenges of leveraging large multi-modal foundational models for this task. We close with discussions of several avenues for future research in this area.
SPEAKER
Shalini De Mello is a Director of Research, New Experiences at NVIDIA, where she leads a team on AI-Mediated Reality and Interaction research. Prior to this, she was a Distinguished Research Scientist in the Learning and Perception Research Group at NVIDIA, which she joined in 2013. Her research interests are in AI, computer vision, and digital humans. She is interested in how AI can re-imagine interactions between humans, and between humans and machines. She has co-authored scores of peer-reviewed publications and patents. Her inventions have led to the creation of several NVIDIA AI products, including NVIDIA DriveIX and Maxine. She received her Doctoral and Master’s degrees in Electrical and Computer Engineering from the University of Texas at Austin.
ABSTRACT
Generative AI and its use by the public demonstrate the challenges with these systems and their ability to generate information about mental health. I discuss what’s going on with Generative AI, its intersection with mental health, and the potential and pitfalls of GenAI for intervening in mental health. With work in collaboration with Logan Stapleton, I present our current work on designing Generative AI-backed training environments for volunteers who provide peer mental health support in suicide crises and future work in the human infrastructure of “redteaming” for dangerous mental illness behaviors for Generative AI systems.
SPEAKER
Logan Stapleton is a PhD student in Computer Science at the University of Minnesota and a member of GroupLens at University of Minnesota, advised by Profs. Haiyi Zhu and Steven Wu. His research studies how people use machine learning models in care work, such as child welfare and suicide prevention. Much of his work focuses on understanding how people are marginalized, discriminated against, or harmed by these technologies. His current work looks at technologies for people who experience suicidality. In his undergraduate days, Logan worked with Prof. Diane Michelfelder on philosophy of technology and critical theory.
ABSTRACT
Generative AI has captured the world’s interest at unprecedented levels. Many enterprises will benefit from Generative AI and the large language models that have fueled its growth, but only if they address a range of challenges customers face, such as hallucinations and random responses. In this talk, we discuss generative AI use cases and key considerations for the enterprise.
SPEAKER
Henrik Ohlsson is Vice President and Chief Data Scientist at C3 AI. He is also an assistant professor at Linkoping University in Sweden, and a visiting professor at the University of California, Berkeley, with both a Master of Science in Electrical Engineering and Applied Physics, and a Ph.D. in Automatic Control from Linkoping University.
ABSTRACT
The emergence of Large Language Models (LLMs) has initiated a transformative wave in diverse research areas, including computational biology. This talk offers an overview of recent advances and explore the potential of LLMs for offering new solutions to complex problems at molecular and cellular scales. Through a discussion of case studies, we outline current challenges and underscore the promising potential of applying LLMs in computational biology.
SPEAKER
Shaoheng Liang is a computer scientist/bioinformatician and a Lane Fellow at Carnegie Mellon University in the Department of Computational Biology, working with Dr. Jian Ma. Before that, he worked at NuProbe USA, Inc., a company innovating cutting-edge sequencing solutions for disease detection. Liang earned his Ph.D. degree from Rice University, Department of Computer Science. Co-advised by Dr. Ken Chen (research advisor) and Dr. Luay Nakhleh (academic advisor), his PhD work was done in Dr. Ken Chen’s Computational Genomics Lab at MD Anderson Cancer Center, where he established wide collaboration with biomedical researchers. Before that, Liang received a Bachelor of Engineering in Electronic Information Science and Technology from Tsinghua University, Department of Electronic Engineering.
SPEAKER
Moderator:
Julia Hockenmaier, University of Illinois Urbana-Champaign
Panelists:
Arindam Banerjee, University of Illinois Urbana-Champaign
Katie Driggs-Campbell, University of Illinois Urbana-Champaign
Mark Hasegawa-Johnson, University of Illinois Urbana-Champaign
Svetlana Lazebnik, University of Illinois Urbana-Champaign
SPEAKER
Kratika Bhagtani (Purdue University), FGSSAT : Unsupervised Fine-Grain Attribution of Unknown Speech Synthesizers Using Transformer Networks
Jiahao Li (Toyota Technological Institute at Chicago), Instant3D: Fast Text-to-3D with Sparse-View Generation and Large Reconstruction Model
Jipeng Lyu (University of Illinois Urbana-Champaign), NeuralEditor: Editing Neural Radiance Fields via Manipulating Point Clouds
Logan Stapleton (University of Minnesota), Using Large Language Models as Virtual Patients for Suicide Prevention Training
Haochen Wang (Toyota Technological Institute at Chicago), Score Jacobian Chaining: Lifting Pretrained 2D Diffusion Models for 3D Generation
Ziyang Xie (University of Illinois Urbana-Champaign), MV-Map: Offboard HD-Map Generation with Multi-view Consistency
Amit Kumar Singh Yadav (Purdue University), DSVAE: Interpretable Disentangled Representation for Synthetic Speech Detection
Xiaocong Yang (University of Illinois Urbana-Champaign), Parameter-Efficient Tuning with Special Token Adaptation
Andy Zhou (University of Illinois Urbana-Champaign), Language Agent Tree Search Unifies Reasoning Acting and Planning in Language Models
Libin Zhu (University of California, San Diego), Quadratic Models for Understanding Neural Network Dynamics
Day 3 (Friday, October 27)
Session Chair:
Morning: Tandy Warnow (University of Illinois Urbana-Champaign)
ABSTRACT
Image (2D) data, with or without text annotations, is abundant compared to data covering 3D shapes and scenes. It is one of the reasons 2D generative modeling has advanced much faster. I discuss two competing approaches to leveraging these advances to lift 2D models into the 3D world (the jury is still out on what the relative merits of these approaches are). The first is optimization-based, using 2D model as a guidance mechanism. The other uses the 2D model as a full-fledged component of the generative process, in conjunction with modern implicit 3D modeling techniques. Joint work with Haochen Wang, Jiahao Li, Xiaodan Du, and others.
SPEAKER
Greg Shakhnarovich is a Professor at Toyota Technological Institute at Chicago (TTIC), a philanthropically-endowed academic computer science institute located on the University of Chicago campus. He received a BSc degree in Mathematics and Computer Science from Hebrew University, Jerusalem, in 1994, an MSc degree in Computer Science from the Technion, Haifa, in 2001, and a PhD degree in Electrical Engineering and Computer Science from MIT in 2005. In 2005-2007, prior to joining TTIC, Greg was a Postdoctoral Research Associate in the Department of Computer Science and the Brain Sciences Program at Brown University. There he worked on computational methods for brain-machine interfaces, with applications in neuro-motor prostheses. Greg is is interested in computational vision and machine learning. His current research is focused on automatic understanding of visual scenes, including recovery of three-dimensional structure and detection and categorization of objects. He is also generally interested in similarity-based, supervised and semi-supervised statistical learning methods.
ABSTRACT
My long-term research goal is to let computers create highly realistic and easily authored 3D virtual worlds and produce new, photorealistic, and physics-plausible visual content. This talk will summarize our group’s two recent directions toward this goal.
I begin by discussing generating realistic movies of physical phenomena in a real-world scene captured by a video — imagine what a children’s park would look like if a flood or a heavy snowstorm hits the neighborhood. Generative models struggle in this setting because of inconsistency and lack of physics feasibility. We believe the key to this question is to integrate physics-informed simulation and generative modeling with neural scene representations. I demonstrate how to integrate the two techniques and simulate coherent, realistic, and physically plausible extreme climate and relighting effects for a visual scene.
I focus on designing generative models that can create a large, realistic, and consistent 3D world. The task is of paramount interest to many applications of vision, graphics, geography, and robotics. Nevertheless, achieving this goal is challenging and goes beyond the capacities of existing 3D generation methods, which fail to scale up to create large scenes. I describe some of our recent efforts in 3D scene generation, including work on learning to generate a realistic LiDAR point cloud of urban driving scenes and our recent work on perpetual 3D world generation. Finally, I give a brief personal outlook on open research topics towards photorealistic 3D modeling and visual content creation.
SPEAKER
Shenlong Wang is an Assistant Professor in the Department of Computer Science at the University of Illinois Urbana-Champaign, specializing in computer vision and robotics. His research focuses on creating a digital replica of the world and simulating realistic new content to train and validate autonomous systems. Shenlong’s past work received the 2020 IROS Best Application Award Finalist and the 2021 CVPR Best Paper Candidate. His contributions to self-driving technologies have led to the filing of over 25 patents. Shenlong has received the Intel and Amazon Research Award, as well as various fellowships from Facebook, Adobe, and the Royal Bank of Canada. He regularly serves as an area chair for conferences in computer vision, robotics, and machine learning.
ABSTRACT
The visual world in which artificial intelligent agents live and perceive is intrinsically open, streaming, and dynamic. However, despite impressive advances in visual learning and perception, state-of- the-art systems are still narrowly applicable, operating within a closed, static world of fixed datasets. In this talk, I discuss our efforts towards developing generalizable and adaptive open-world perception and learning systems. Our key insight is to introduce a mental model with hallucination ability – creating internal imaginations of scenes, objects, and their variations and dynamics not actually present to the senses. I focus on how to integrate such an intrinsic mental model with extrinsic task-oriented models and construct a corresponding closed-loop feedback system. I demonstrate the potential of this framework for scaling up open-world, in-the-wild perception in application domains such as transportation, robotics, geospatial intelligence, and healthcare.
SPEAKER
Yuxiong Wang is an Assistant Professor in the Department of Computer Science at the University of Illinois Urbana-Champaign. He is also affiliated with the National Center for Supercomputing Applications (NCSA). He received a Ph.D. in robotics from Carnegie Mellon University. His research interests lie in computer vision, machine learning, and robotics, with a particular focus on few-shot learning, meta-learning, open-world learning, streaming perception, and generative modeling. He is a recipient of awards including the Amazon Faculty Research Award, the ECCV Best Paper Honorable Mention Award, and the CVPR Best Paper Award Finalists.
VENUE
National Center for Supercomputing Applications
University of Illinois Urbana-Champaign
Urbana, Illinois 61801
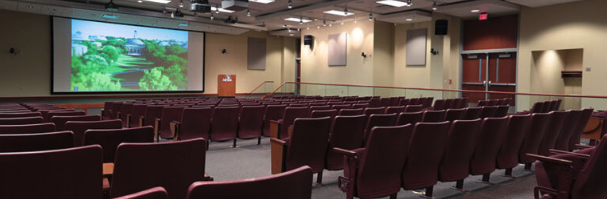
LOCAL ACCOMMODATIONS
Hampton Inn Champaign/Urbana, 1200 West University Avenue, Urbana, Illinois 61801
TownePlace Suites Champaign Urbana/Campustown, 603 South Sixth Street, Champaign, Illinois 61820
All times are Central Time. Event information subject to change.